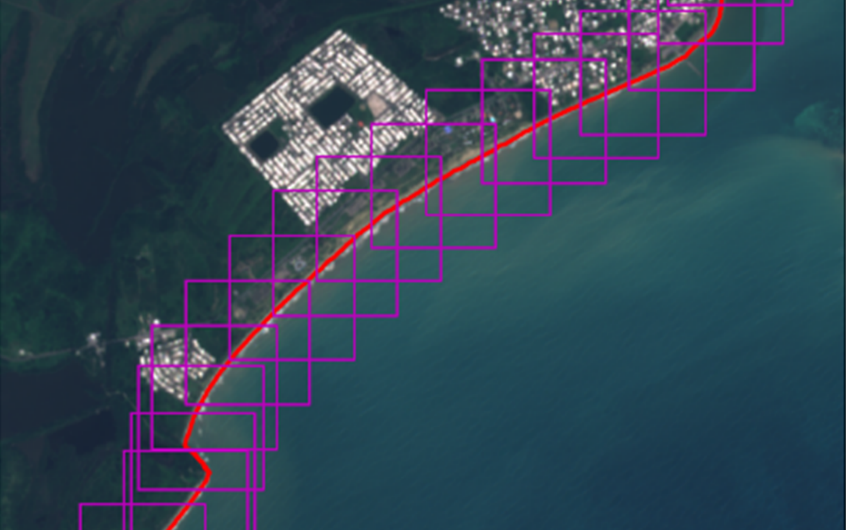
A new dataset of satellite images for deep learning-based…
Methodology: The usage of coastline data published in scientific projects is explored for the generation of a dataset of labelled satellite images for sea-land segmentation/coastline detection tasks. Sentinel-2 Level-1C images are considered for the dataset. The Sentinel-2 mission provides high-resolution satellite images with 13 spectral bands [2]. Four bands have spatial resolution of 10 m, six bands have resolution of 20 m, and three bands have resolution of 60 m. All continental land and coastal waters up to 20 km from the shore are covered by the mission, with a revisit time of 5 days.
The coastline data used for labelling satellite images is taken from the NOAA Continually Updated Shoreline Product (CUSP) project [3]. This dataset contains the coastline of the USA and is continually updated. The coastline is split in short segments annotated with additional information such as the date and type (e.g., satellite or aerial) of data used for the coastline extraction and the type of coast. Given the availability of these additional information and the high resolution of coastlines, this dataset has been chosen for our work.
CUSP data must be filtered to obtain valid samples for the task at hand. Coastline of Alaska are excluded, since it contains regions covered by ice (we focus on exposed land). Only observations made later than December 2016 are considered, (Sentinel-2 Level-1C products availability). Only the following types of coasts are considered (CUSP nomenclature):
“Man-made.Rip Rap” + “Natural.Great Lake Or Lake Or Pond” + “Natural.Mean High Water”
Excluded types of coasts were e.g., rivers and jetties, that would have led to images containing most of the Sentinel-2 pixels of a single class.
The Sentinel-2 tiles containing the selected coastline segments has been identified by querying the PEPS CNES platform. Only results of the query characterized by a cloud cover lesser than 3% are considered, and, among them, that with the nearest date to the observation date of the segment are chosen. The maximum allowed temporal distance between the segment date and the Sentinel tile date was set to 30 days. At the end of the procedure, 155 Sentinel tiles were selected.
The coastline segments are projected to the ortho-images plane, and 64×64 squared tiles are extracted following the coastline. The position of the extracted tiles is chosen so that the coastline intersects each tile in two points, and 50% of overlap between consecutive tiles was used to maximize the quantity of unique pixels in the dataset (see Fig. 1). Each extracted tile is further processed to create the relative binary segmented label. CUSP provides only the coastline and gives no information which of the two regions defined by the coastline is sea or land. The water bodies detection based on the band 2/band 11 ratio is used for this purpose. Besides, tiles with at least 90% of pixels detected as water on one side, and at least 90% of pixels as non-water on the other side are labelled; the other are discarded. This method implies a verification of CUSP data (Fig. 2).
Conclusions: The presented method proved to be effectively usable for generating datasets of labelled satellite images. Its clear advantage is that it allows reusing high-quality coastline data created by experts, to label satellite images of different types (multispectral images, SAR images) and acquired from different sources. The effectiveness of the method has been successfully demonstrated using NOAA CUSP coastline data and Sentinel-2 multispectral images, but the procedure can be replicated using other coastline data and satellite images.
References:
[1] M. Scarpetta, M. Spadavecchia, V. I. D’Alessandro, L. D. Palma and N. Giaquinto, “A new dataset of satellite images for deep learning-based coastline measurement,” 2022 IEEE International Conference on Metrology for Extended Reality, Artificial Intelligence and Neural Engineering (MetroXRAINE), 2022, pp. 635-640, doi: 10.1109/MetroXRAINE54828.2022.9967574.[2] https://sentinel.esa.int/web/sentinel/missions/sentinel-2[3] https://shoreline.noaa.gov/data/datasheets/cusp.html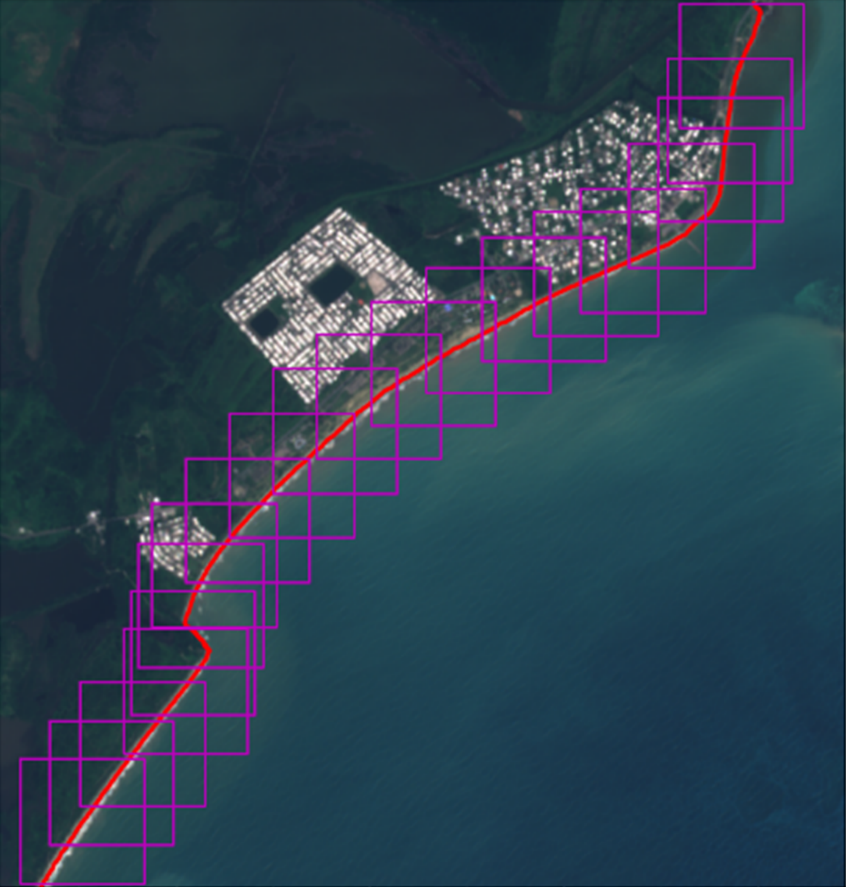
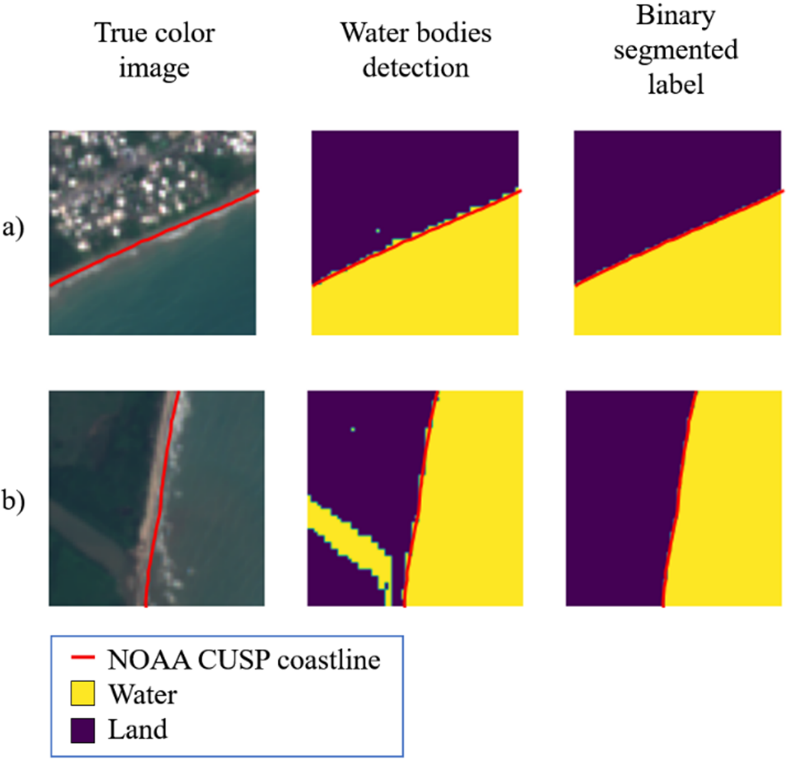